Olivier Elemento, Director of the Englander Institute for Precision Medicine
Summary
- Tissue or organ-level changes in gene expression patterns often precede the onset or progression of disease
- Single-cell sequencing data overlaid on maps of tissue architecture, termed spatial transcriptomics, is a powerful method yet requires time-consuming manual annotation and the risk of user bias
- The Elemento lab has been pioneering a computational method to identify and quantify organ-specific anatomical domains from biological images without any previous knowledge of disease state
- By combining this multiplexed imaging framework with single-cell phenotypic data (e.g. cell morphology, gene expression, cell surface markers), the EIPM has a powerful tool which can yield therapeutically actionable insights into disease states and drug mechanisms
Technical Overview
- Advancements in spatial transcriptomics are providing researchers the opportunity to directly characterize cellular phenotypes and interactions in intact, heterogeneous tissues
- However, the technology has been unable to move beyond “cell-centric” insights to uncover organizing principles to tissue architecture and organ-specific tissue pathophysiology
- Dr. Elemento and the EIPM have developed an accurate, unsupervised method to discover and quantify “microanatomical tissue structures” using multiplexed histopathology images
- The deep learning model provides novel insights on cell morphology and gene expression, coupled to physical proximity data at a whole-organ scale in both healthy and diseased tissues
Market Opportunity
- Advancements in spatial transcriptomics are providing researchers the opportunity to directly characterize cellular phenotypes and interactions in intact, heterogeneous tissues
- However, the technology has been unable to move beyond “cell-centric” insights to uncover organizing principles to tissue architecture and organ-specific tissue pathophysiology
- Dr. Elemento and the EIPM have developed an accurate, unsupervised method to discover and quantify “microanatomical tissue structures” using multiplexed histopathology images
- The deep learning model provides novel insights on cell morphology and gene expression, coupled to physical proximity data at a whole-organ scale in both healthy and diseased tissues
Partnering Opportunity
Weill Cornell Medicine is seeking a partner with an interest in leveraging EIPM’s comprehensive patient biobank and deep learning model to improve biomarker strategy and clinical development insight
Supporting Data / Figures
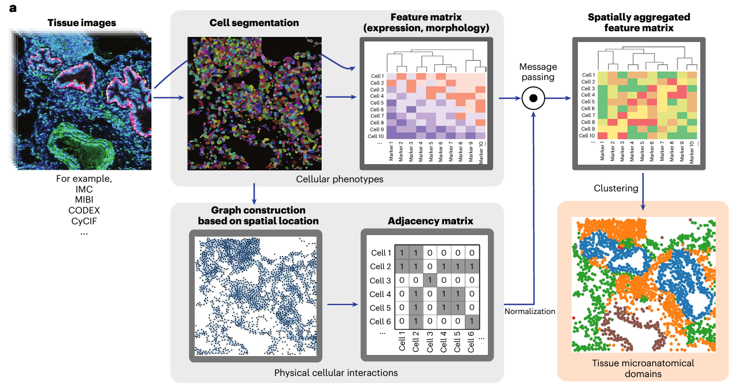
Figure 1: Overview of UTAG, unsupervised discovery of tissue architecture with graphs, deep learning model for biomarker discovery (Kim 2024 Nature).
Contact Information
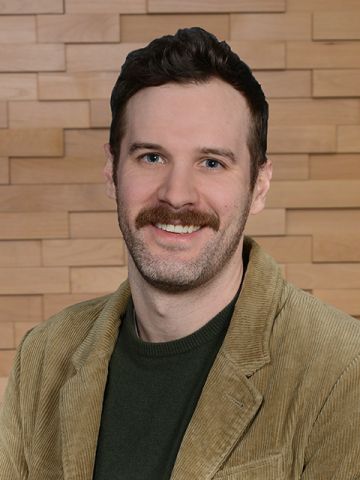
For additional information please contact
James Bellush
Manager, Scientific Scouting
Phone: (646) 962-7080
Email: james.bellush@cornell.edu