Principal Investigator:
Peter Yan
Background & Unmet Need
- Seizures occur in up to 50% of critically ill patients with altered consciousness, and >80% present with no obvious clinical sign of motor activity
- These non-convulsive seizures (NCS) have associated high morbidity and mortality in critically ill patients, and warrant prompt detection and treatment
- A continuous electroencephalogram (cEEG) is the gold standard for diagnosing NCS but is resource intensive and only reviewed intermittently (often 2-3 times daily) rather than continuously monitored
- Quantitative EEG (qEEG) tools apply digital signal processing techniques to facilitate cEEG interpretation, but require lengthy clinician training and are limited in types of seizures detected
- Unmet Need: Access to rapid, accurate, and automated continuous EEG seizure detection
Technology Overview
- The Technology: Method for continuous automated seizure detection based on artificial neural network recognition of seizure patterns on a novel spectrographic display
- The method introduces the median power spectrogram (MPS), a novel qEEG spectrographic display which can consolidate multiple EEG channels into a single channel display and optimize temporal and frequency resolution, resulting in well visualized seizures
- Seizures appear as characteristic sloped harmonic bands on MPS that are visually distinct and easily identified with minimal clinician training (~5 min)
- A convolutional neural network (CNN) can be trained to recognize seizure patterns on the MPS and can automatically detect seizures in a continuous fashion
- PoC Data: The CNN models detected seizures with 80–90% sensitivity and specificity, on both adult and pediatric cohorts
Technology Applications
- Continuous seizure telemetry monitoring at bedside for critically ill patients that can automatically alert the bedside clinicians to enable faster intervention
- A visual bedside display where seizures are easily recognized, and the clinician has the option to visually confirm the automated seizure detection
- A visual display for the neurophysiologist that supplements and expedites traditional EEG analysis
Technology Advantages
- Concise EEG visualization where seizures are easily recognizable, requiring less clinician training
- Rapid, accurate, and continuous automated seizure detection, in a real-time telemetry fashion, at the beside that enables faster interventions
- A bedside display easily interpreted by a bedside clinician who can visually confirm automated seizure detection, and monitor response to treatment
Publications
Resources
Intellectual Property
Patents
- US Patent Application Filed: US20220211318A1. "Median power spectrographic images and detection of seizure." Priority Date Apr 29, 2019.
Cornell Reference
- 7812
Contact Information
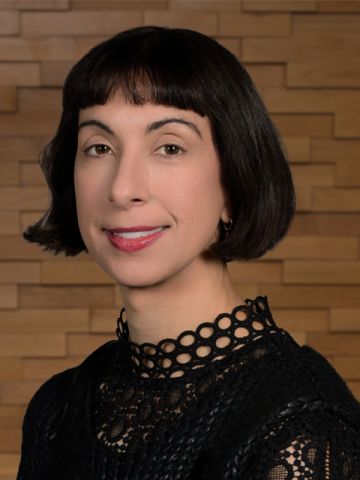
For additional information please contact
Louise Sarup
Associate Director, Business Development and Licensing
Phone: (646) 962-3523
Email: lss248@cornell.edu