Bruce S. Kristal
Technology Overview
Current neural networks can find unknown relationships from highly complex and/or non-linear data input and their predictive outcomes. When data is very limited or even absent, a machine generated algorithm may produce random behavior and poor network predictions. External or human input allows the network to adjust individual nodes and connections, and combine this with machine generated algorithms to strengthen the predictive output.
Dr. Bruce Kristal and colleagues at Weill Cornell Medicine devised a method of utilizing human guesstimates to initialize nodes in a machine learning network. The host machine will propose at least one question on a predetermined topic to multiple users and use those answers to strengthen the value of the outcome. By giving questions with or without a choice of results to experts in a field or others with domain expertise (and potentially weighting answers to perceived expertise, e.g., professional rank), the responses can be fed into the network and the host network can adjust connections between the nodes to generate a more accurate responses. By analyzing external input and machine generated algorithms, a hybrid training set is produced that can be used to improve prediction of outcomes and/or recognition of patterns despite weak or absent training exemplars.
By utilizing this method, a network can generate decisions using additional synthetic (human or human-machine generated) examples to reduce the current necessity of training the network with known input and outcome data required to achieve high accuracy. Input which introduces human knowledge, wisdom and insight into network connections, including interrelationships between variables of interest provides the framework for predictions across many areas, including speech and handwriting recognition, stock analysis, drug development, event wagering, medical diagnosis, detection of credit card fraud and classification of DNA sequences.
Resources
Intellectual Property
Patents
- Issued US Patents: System and Method to Enable Training a Machine Learning Network in the Presence of Weak or Absent Training Exemplars (US Patent 8,095,480 and US Patent 8,838,511)
Cornell Reference
- 3697
Contact Information
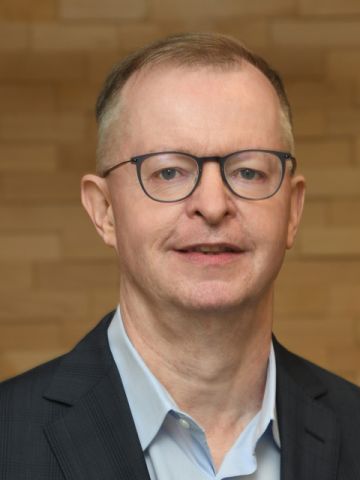
For additional information please contact
Brian Kelly
Director, Business Development and Licensing
Phone: (646) 962-7041
Email: bjk44@cornell.edu