Principal Investigator:
Iman Hajirasouliha, Associate Professor of Physiology and Biophysics
Background & Unmet Need
- The success of in vitro fertilization (IVF) is limited by challenges in selecting the most viable embryos
- As women age, the incidence of euploid embryos (normal chromosome number) decreases while that of aneuploids (embryos with chromosomal abnormalities that may cause miscarriages or birth defects) increases
- The current standard methods for embryo selection, morphological quality and morphokinetic analyses, suffer from intra- and inter- observer variability
- A third method, pre-implantation genetic testing for aneuploidies (PGT-A), is less variable but has its own notable limitations, including invasiveness and cost
- Machine-learning approaches for assessment of embryo quality based on morphology have not demonstrated a clear benefit over current methods
- Unmet Need: A non-invasive, reliable, and high throughput method to predict ploidy of candidate embryos prior to implantation in order to increase the success of IVF
Technology Overview
- The Technology: A machine-learning based method, called STORK-A, to non-invasively predict embryo ploidy status
- Uses time-lapse microscopy images of embryos and clinical information (e.g., maternal age & morphological assessments) as inputs and outputs a probability of euploid vs aneuploid for each embryo
- PoC Data: The STORK-A algorithm was trained using a dataset of images and clinical information for >10k embryos with confirmed ploidy status and was then also tested on two independent external datasets
- STORK-A classified embryo ploidy status with accuracies of ~70% for both the training and independent datasets
- IVF transferred embryos that were predicted to be euploid by STORK-A exhibited a livebirth rate of 48%, which is very similar to that of the transferred embryos classified as euploid by PGT-A (49%)
Technology Applications
- Supplement traditional methods of embryo selection and prioritization by assigning ploidy predictions to embryos in a high throughput and unbiased manner
- Assist embryologists in determining on which embryos the more invasive and costly PGT-A should be performed in IVF cases that are complex and/or unlikely to be successful (advanced maternal age, low embryo count, etc.)
Technology Advantages
- Not subject to observer variability
- Non-invasive and less expensive than PGT-A
- High-throughput and can easily be adopted by fertility clinics for use in the IVF process
- Machine-learning algorithm has ability to improve accuracy as more image data is accrued
- Accuracy can be further increased by integrating with spatiotemporal data (video)
Figure 1: Example STORK-A interface and ploidy predictions for use in clinical settings as a support tool for embryologists.
Publications
Intellectual Property
Patents
- PCT Application Filed
Cornell Reference
- 10145
Contact Information
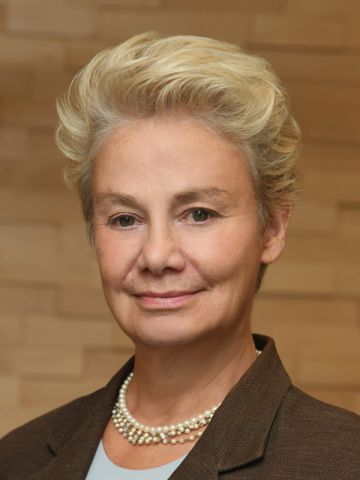
For additional information please contact
Donna Rounds
Associate Director, Business Development and Licensing
Phone: (646) 962-7044
Email: djr296@cornell.edu